Research topics

Neuromorphic computing
Spiking Neural Networks (SNN) are artificial neural networks that include time in their operational process, whose nodes are integrated-and-fire neuron models and in which learning takes place on the basis of biologically realistic learning rules.
The tasks on which the research activity is focused are:
- selectivity of the directionality of movement in retinal inspired spiking networks,
- unsupervised pattern recognition (classification of images) in shallow and deep SNN,
- spatial navigation with hippocampal inspired neural networks.
Inferential multi-agent networks
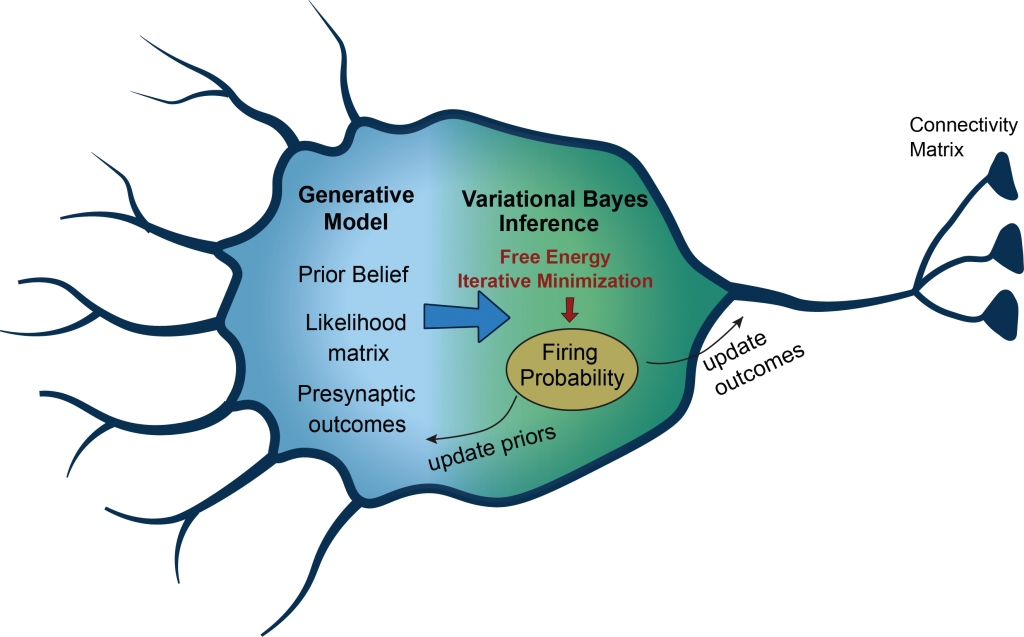
Under the theoretical framework of predictive coding and active inference, brain function is described as a dynamical process that continuously updates a generative model of the world to predict incoming sensory data. This inference process is thought to operate at multiple scales and we proposed a microcircuit model were single neurons are modelled as inferring agents.
We applied this framework in the context of a cerebellar circuit performing the task of delayed eyeblink conditioning. Neurons and synapses adjusted their activity to minimize their prediction error and were able to effectively expressed associative learning.
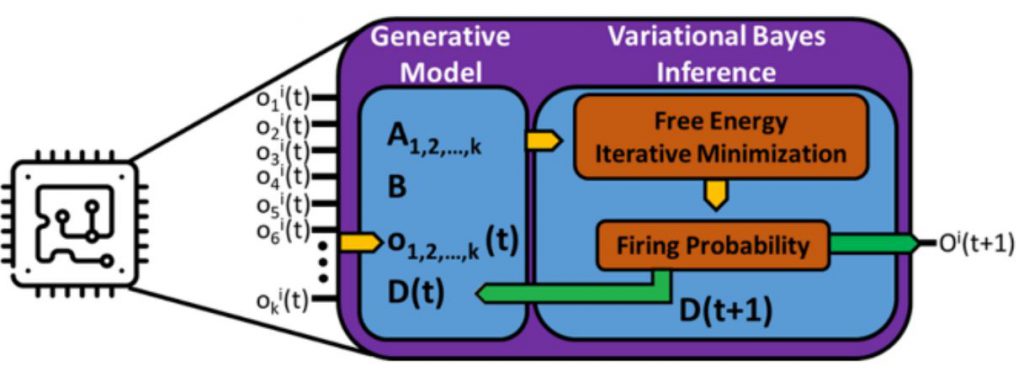
We also replicated this circuit in hardware via a low-power microcontrollers which emulated the behavior of the inferring neurons.
Large scale modelling
We are working at the development of a large scale model of the murine and human hippocampus through realistic modelling strategy, exploiting physiological, morphological and anatomical data. We proposed a novel method to implement plausible connectivity by using the geometrical probability volumes associated with axons and dendrites. We have assessed the validity of our model for the mouse hippocampus CA1 area, and we plan to extend this modelling strategy to the entire hippocampal formation.
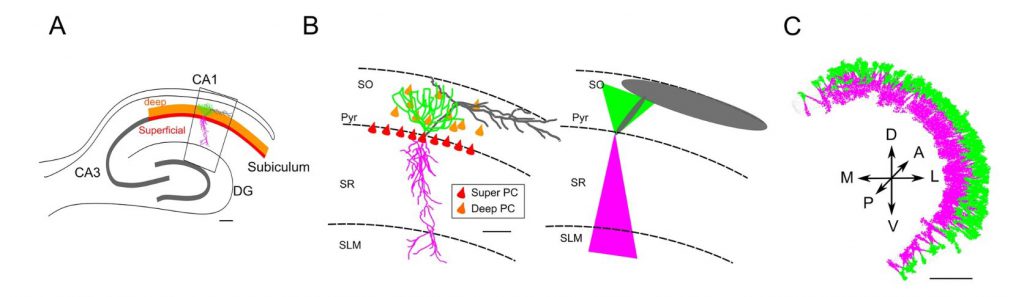